
appears non-uniform) but describing the relative likelihood of different underlying processes generating different types of concentration. The power of this methodology lies in not just identifying if the data is concentrated in some way (i.e.
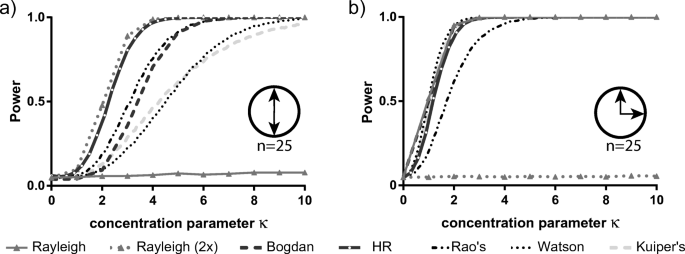
Their approach involved fitting a suite of ten potential models to a sample of circular data and then using the Akaike Information Criterion (AIC Aho et al., 2014 Akaike, 1973) to compare how well the data fitted the different models. Recently, Fitak and Johnsen (2017) presented how model-based approaches can be used for circular data. Such statistical approaches could also be applied to circular data, where null-hypothesis testing remains the dominant statistical approach. In addition, simulation approaches have also been proven useful to correct for type I error rate inflation due to rounded data ( Landler et al., 2019a).įor linear data, there has been a broadening of statistical approaches over the last 20 years, with reduced emphasis on null-hypothesis testing and increased use of Bayesian and model-selection techniques. One such test is the Hermans-Rasson test, which has high statistical power over a broad range of situations and is considerably more powerful than the Rayleigh test for certain multimodally clustered distributions ( Landler et al., 2019b). However, more computationally-demanding simulation-based approaches have been developed more recently ( Pewsey et al., 2013). Other tests that are often used in circular statistics and are known to detect unimodal and multimodal departures from uniformity include the Rao's spacing test, the Watson test and the Kuiper's test (see Batschelet, 1981 for an overview). Von Mises and projected normal alternative) from circular uniformity ( Bhattacharyya and Johnson, 1969 Landler et al., 2018 Mardia and Jupp, 2009).
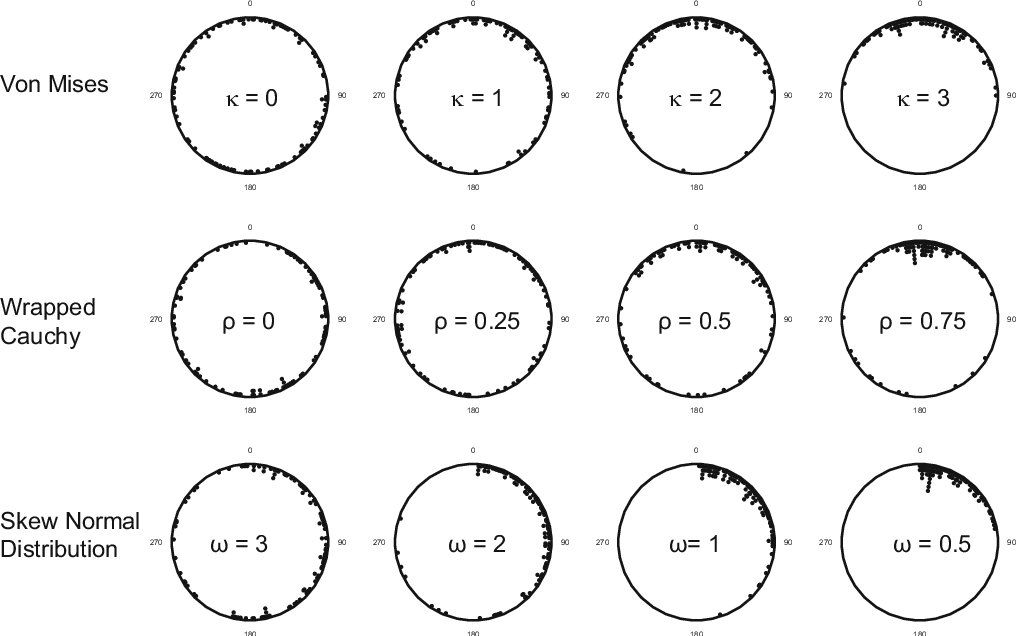
Although it was one of the earliest circular statistical techniques, the Rayleigh test is the most powerful test for detecting some unimodal departures (e.g. Overwhelmingly the most common approach to testing this null hypothesis is the Rayleigh test ( Rayleigh, 1880).
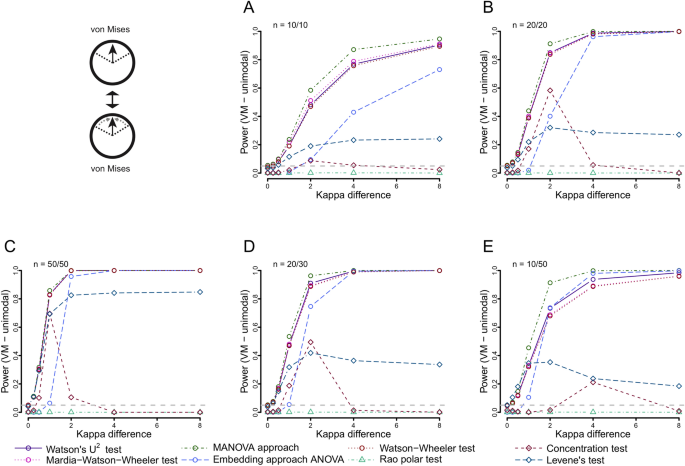
The most common statistical exploration of circular data involves testing the null hypothesis that the data are uniformly distributed across all possible values around the circle (versus some form of concentration). annual cycles and circadian rhythms) and treatment of such data has been reviewed in several monographs ( Batschelet, 1981 Fisher, 1995 Ley and Verdebout, 2017 Mardia and Jupp, 2009 Pewsey et al., 2013). animal orientation) to time-dependent data (e.g. Circular data are common in natural sciences, ranging from directional (e.g.
